machine learning features and targets
Features are usually numeric but structural features such as strings and graphs are used in. Intro part 1 2.
The load_iris function would return numpy arrays ie does not have column headers instead of pandas DataFrame unless the argument as_frameTrue is specified.
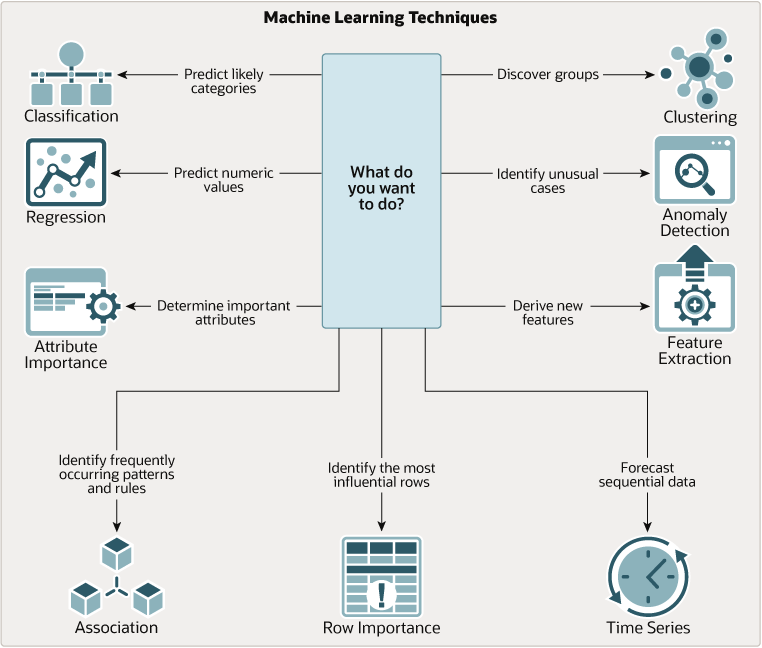
. When I also draw a scatter of this data the low correlation is also clear so that for any value of a specific feature is mapped to all possible values of the target. The learning algorithm finds patterns in the training data such that the input parameters correspond to the target. Choosing informative discriminating and independent features is a crucial element of effective algorithms in pattern recognition classification and regression.
Machine-learning startups in the. Overfitting with Target Encoding. This feature selection process takes a bigger role in machine learning problems to solve the complexity in it.
Machine learning services from Oracle make it easier to build train deploy and manage custom learning models. The output of the training process is a machine learning model which you can. When I analysed the correlation between each feature and the target restNum using Orange Tool I noticed that there is always low correlation between them and the target.
A compute target is a designated compute resource or environment where you run your training script or host your service deployment. The goal of this process is for the model to learn a pattern or mapping between these inputs and the target variable so that given new data where the target is unknown the model can accurately predict the target variable. The image above contains a snippet of data from a public dataset with information about passengers on the ill-fated Titanic maiden voyage.
A supervised machine learning algorithm uses historical data to learn patterns and uncover relationships between other features of your dataset and the target. A machine learning model maps a set of data inputs known as features to a predictor or target variable. Using compute targets makes it easy for you to later change your compute environment without having to change your code.
A feature is a measurable property of the object youre trying to analyze. As companies valuations plummet amid the market downturn insiders and VCs predict an MA wave. For example you can see the.
In datasets features appear as columns. Feature selection methods are intended to reduce the number of input variables to those that are believed to be most useful to a model in order to predict the target variable. True outcome of the target.
Each feature or column represents a measurable piece of data that can be. Up to 50 cash back We almost have features and targets that are machine-learning ready -- we have features from current price changes 5d_close_pct and indicators moving averages and RSI and we created targets of future price changes 5d_close_future_pctNow we need to break these up into separate numpy arrays so we can feed them into machine learning. The target variable will vary depending on the business goal and available data.
This location might be your local machine or a cloud-based compute resource. Supporting machine learning services provide. Separating the features and targets is convenient for training a scikit-learn model but combining them would be helpful for visualization.
An example of target encoding is shown in the picture below. The target variable of a dataset is the feature of a dataset about which you want to gain a deeper understanding. For instance Seattle can be replaced with average of salary target variable of all datapoints where city is Seattle.
Up to 55 cash back Create features and targets. Machine learning is unique within the field of artificial intelligence because it has triggered the largest real-life impacts for business. Also we pass return_X_yTrue to the function so only the machine learning features and targets are returned rather than some metadata such as the description of the dataset.
In machine learning and pattern recognition a feature is an individual measurable property or characteristic of a phenomenon. Target encoding involves replacing a categorical feature with average target value of all data points belonging to the category. Feature selection is primarily focused on removing non-informative or redundant predictors from the model.
We almost have features and targets that are machine-learning ready -- we have features from current price changes 5d_close_pct and indicators moving averages and RSI and we created targets of future price changes 5d_close_future_pct. Due to this machine learning is often considered separate from AI which focuses more on developing systems to perform intelligent things. Final output you are trying to predict also know as y.
It can be categorical sick vs non-sick or continuous price of a house. In supervised learning the target labels are known for the trainining dataset but not for the test. What is a Feature Variable in Machine Learning.
Now we need to break these up into separate numpy arrays so we can. A supervised machine learning algorithm uses historical data to learn patterns and uncover relationships between other features of your dataset and the target. In supervised learning the target labels are known for the trainining dataset but not for the test.
The target variable will vary depending on the business goal and available data. These services deliver data science capabilities with support from favorite open source libraries and tools or through in-database machine learning and direct access to cleansed data.
A Single Neuron In A Neural Network Artificial Intelligence Electroica Blog Deep Learning Artificial Neural Network Machine Learning
What Are Feature Variables In Machine Learning Datarobot Ai Wiki
How To Choose A Feature Selection Method For Machine Learning
Pin On Artificial Intelligence
A 6 Step Field Guide For Building Machine Learning Projects By Daniel Bourke Towards Data Science
What Are Feature Variables In Machine Learning Datarobot Ai Wiki
Supervised And Unsupervised Machine Learning Algorithms
Understanding Machinelearning Learn How You Can Modernize Your Data Warehouse With Apache Hadoop View Machine Learning Deep Learning Big Data Technologies
Target Variable Datarobot Artificial Intelligence Wiki
How To Choose A Feature Selection Method For Machine Learning
Data Science Free Resources Infographics Posts Whitepapers Machine Learning Artificial Intelligence Data Science Learning Machine Learning
How To Choose A Feature Selection Method For Machine Learning
Feature Engineering Secret To Data Science Success Data Science Feature Extraction Science
Oracle Machine Learning Basics
How To Compare Machine Learning Models And Algorithms Neptune Ai
A 6 Step Field Guide For Building Machine Learning Projects By Daniel Bourke Towards Data Science